TLDR: Generative AI is a type of artificial intelligence (AI) that creates realistic content like images, text, and videos. It works by using a neural network to learn from a dataset and then generate new content based on what it “learned.” However, generative AI can cause serious harm if not used responsibly, such as privacy risks, bias, security threats, lack of transparency, environmental costs, and more. To mitigate these risks and get the most out of this exciting technology, businesses must implement Responsible AI (RAI) frameworks grounded in leading standards and best practices.
AI has rapidly advanced in the past few years, leading to new applications and opportunities. Generative AI is one such application that has gained significant attention, in the form of chatbots like ChatGPT or deepfake filters that look eerily real. In this blog post, we will explain what generative AI is, how it works, what risks it presents, and what you can do to shape the responsibility of generative AI in our society.
What is Generative AI?
With the recent surge in interest in AI surrounding the launch of OpenAI’s chatbot ChatGPT, there has been a lot of talk about generative AI. Generative AI is a type of AI that “learns” from tons of data and then creates new data or content. It can generate anything from text, images, videos, and even music, with the goal of creating something similar to what a human artist might create. For example, Bard, ChatGPT, and CoPilot are examples of large language models, or LLMs, while other types of generative AI generate art, such as Midjourney or NightCafe.
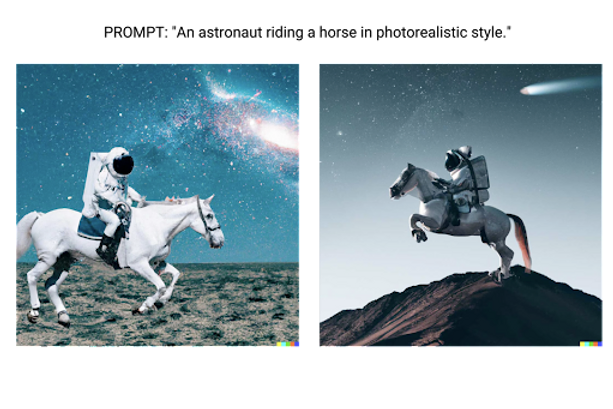
Images generated by OpenAI’s DALL-E.
How Does Generative AI Work?
Generative AI works by using a technique called deep learning. Deep learning is a subset of machine learning that uses artificial neural networks to learn from data. Generative AI models use a specific type of neural network called a Generative Adversarial Network (GAN). A GAN consists of two networks, a generator, and a discriminator.
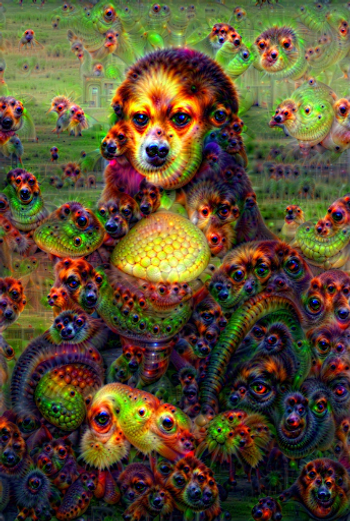
The Mona Lisa generated with Google’s DeepDream effect
The generator network generates new content by learning patterns and trends from a dataset. It then creates new content by altering and combining existing data in the dataset. The discriminator network evaluates the new content and determines whether it is real or generated. The generator network then uses this feedback to create better and more convincing content.
In short, generative AI uses machine learning algorithms to learn patterns and trends from a dataset. It then generates new exciting content based on that learning.
Examples of Generative AI
Several companies have designed generative AI models for public use. One such company is OpenAI, which released its AI system called GPT 3 or ChatGPT in November 2019. ChatGPT can generate human-like text based on a given prompt. For example, given a prompt like “Write an essay about the importance of recycling,” ChatGPT can generate a convincing essay that reads like it was written by a human.
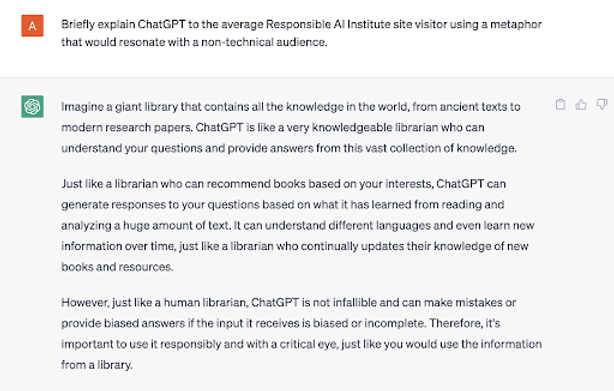
Example output of OpenAI’s ChatGPT
NVIDIA is another company that has designed its own generative AI model called StyleGAN2, which can generate high-quality images of people that do not exist in real life. This technology has significant implications for the entertainment and advertising industries.
Other examples of Generative AI
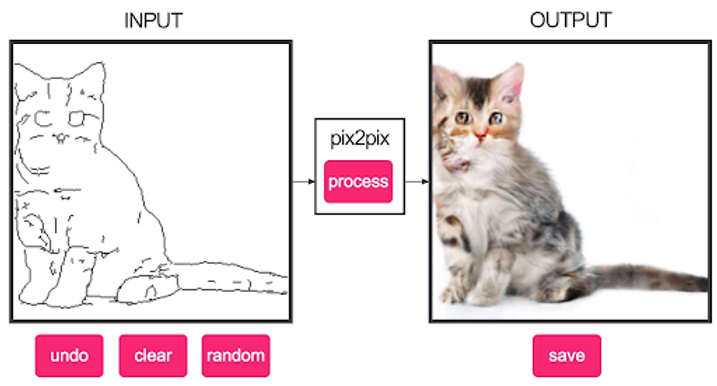
Auto-filled kitten generated with Pix2Pix.
- MuseNet: A deep learning model developed by OpenAI that can generate music in a variety of genres and styles.
- GROVER: A generative model developed by the Allen Institute for AI that can generate realistic news articles, reviews, and social media posts.
- DeepDream: A neural network visualization technique developed by Google that can generate surreal and psychedelic images from ordinary photographs.
- Pix2Pix: A conditional generative model that can translate images from one domain to another, such as turning a sketch into a realistic image or converting day-time images into night-time ones.
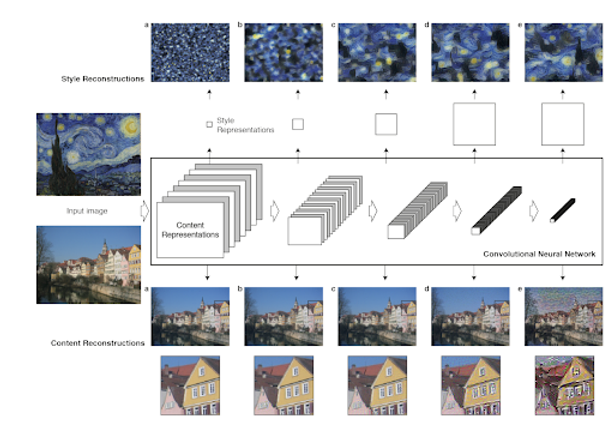
Neural Style Transfer method from “A Neural Algorithm of Artistic Style” by Leon A. Gatys et al.
In this figure, we see an exercise in mimicking human artistic creativity through generative AI. This is an example of how a convolutional neural network, a type of deep learning model, can analyze a given picture (e.g., a street with houses) and a given artistic style (e.g., Van Gogh’s Starry Night) to learn the style and apply it to the picture through multiple layers of processing.
Risks of Generative AI
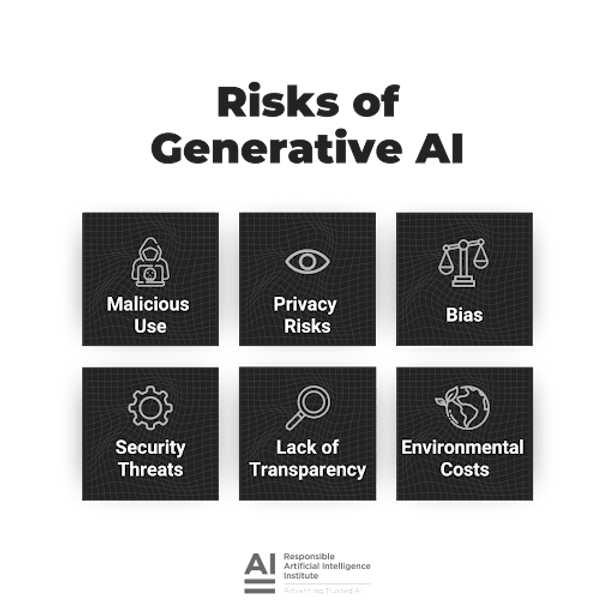
Generative AI technology can cause harm if not used responsibly. One risk is that it can be used to mislead people with fake videos and images, such as deepfakes used to scam or misrepresent individuals. Generative AI can also cause harm with biased outputs if it’s only trained on information from certain groups, which can lead to unfair and unrepresentative outcomes. For example, when prompted to describe or depict a “professional person in the workplace,” an AI system that was trained on biased data might omit photos of women, particularly women of color.
Furthermore, AI systems can also generate factually inaccurate outputs, even making up or “hallucinating” research reports, laws, or historical events in their outputs. This hallucination occurs when an AI system learns from data and produces its own new, plausible-seeming but fabricated information. This can occur due to data quality and mitigation issues such as biased or limited training data and model overfitting in response to the data.
There are also other risks associated with generative AI, such as security problems. For example, generative AI can rely on large-scale datasets that hold private information about individuals that can be elicited through prompts. This also poses intellectual property concerns in terms of both the inputs and outputs to the AI. For example, a recent study of over 10,000 employees found that 15 percent of employees input company data into ChatGPT putting their company at risk of a security breach.
Security breaches can happen due to a generative AI system’s vulnerability to threats such as model theft, data poisoning, and adversarial attacks. Another risk is a lack of transparency in the AI’s decision-making processes, which can confuse both users and developers on a system’s outputs and blur the lines of legal liability.
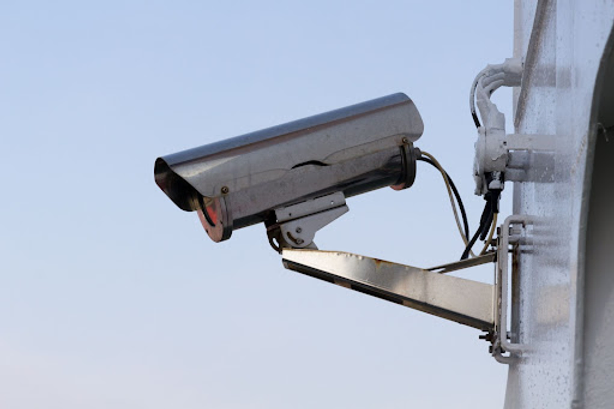
How Can We Address These Risks?
We can all play a role in helping reduce the harm caused by generative AI by taking certain steps. Here is an overview of what we can do to promote responsibility in generative AI based on the above risks.
What Businesses Can Do
Businesses have an obligation to develop and utilize AI systems, including generative AI, responsibly. Moreover, responsible AI is good for business. A study by Edelman showed that 81% of consumers prefer purchasing from companies that prioritize data privacy and security. Furthermore, research by PwC found that 60% of consumers are more likely to trust companies that are transparent about their AI use. According to a report by Deloitte, a trustworthy AI approach “can mitigate risks that might otherwise reduce confidence in AI systems and stifle innovation in this critical sector while focusing investment on beneficial applications of AI that can lead to economic growth and improved health, safety, and well-being.”
So how can businesses ensure they’re mitigating the risk of their own generative AI products or third-party generative AI that’s used for business purposes?
Public responsible AI principles and frameworks are a best practice and demonstrate a strong commitment to AI responsibility to consumers and investors alike. Generative AI should be a part of an organization’s broader Responsible AI frameworks that guide how AI is designed, developed, and deployed. These frameworks provide guidelines for managing risks associated with AI deployment and can consist of generative AI principles, risk assessments, training, and testing.
From these frameworks and principles, businesses can and should issue enterprise-wide generative AI guidance and policies. In doing so, it can clarify the role of generative AI at the organization and share best practices in operating generative AI, such as fact-checking, preserving sensitive data and intellectual property, etc.
For example, leaders in this space have done the following:
- Shared enterprise-wide generative AI guidance on best practices, regulations, and intellectual property considerations
- Offered lunch-and-learns, Slack channels, intranet pages, and repositories for employee discussion and knowledge-sharing about generative AI
- Established an internal Responsible AI review board with external subject matter experts to ensure the company’s use of AI aligns with principles and objectives
- Developed procedures to track and research leading AI and generative AI technologies as well as relevant regulations, best practices, and mitigation strategies
- Built AI-specific procurement/vendor evaluation procedures to ensure the responsible acquisition and evaluation of third-party AI systems
- Empowered employees to pursue AI-related professional development opportunities, including training, pilot projects, and contributions to relevant standards and open-source technology research
- Pursued Responsible AI certification of their AI systems in alignment with national accreditation standards and leading best practices
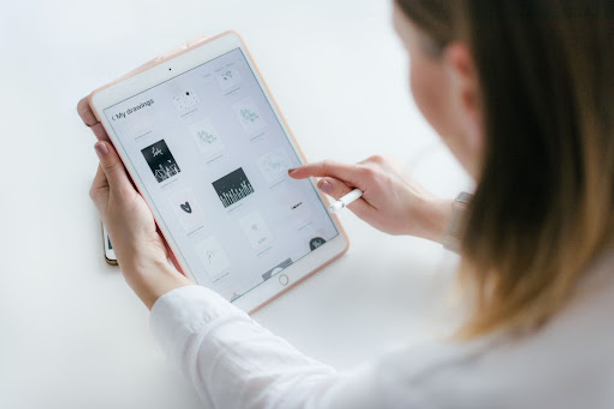
What Consumers Can Do
For consumers, awareness of the risks of generative AI is a critical first step. That way, consumers can make informed decisions and protect themselves from malicious uses of generative AI, like hacking and data theft. To enhance your AI literacy, it’s a good idea to educate yourself on fraud warning signs, the benefits and drawbacks of specific technologies, telltale signs of AI-generated images, and more.
Based on this research, you can take steps to protect your personal information with good personal data protection hygiene. This could include installing a password manager, using a VPN, adding a browser extension to prevent tracking cookies from collecting browsing history, and doing regular password audits. When interacting with generative AI, be careful to avoid putting any confidential or personal information into the AI system as it may use that data to train on or as an output.
Additionally, consumers can influence the generative AI market in their buying choices. You can choose to buy or do business with generative AI tools with strong reputations and commitments to the responsible use of AI. To research this, you can look for a seal of approval from national AI standards bodies, like a Responsible AI Certification (coming soon), or other independent reviews of the business. For example, Consumer Reports will test and rate products against their principles, sharing back information about which apps don’t sell personal data, for example.
Lastly, consumers have the power to influence the actions of both regulators and businesses by using their voices to advocate for their beliefs and rights. You can let your elected representatives know that you care about specific AI regulations, and pressure them to take action to ensure that generative AI tools follow global best practices.
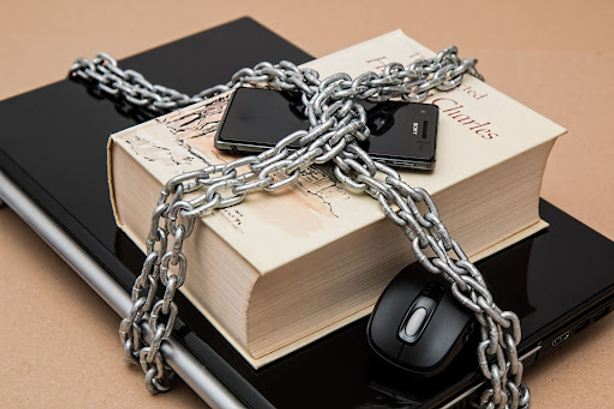
What Regulators Can Do
Regulators play a vital role in mitigating the risks of generative AI by setting clear standards for responsible AI development and use. Penalties can be imposed on companies that violate these requirements and responsible guidelines. They can ensure that AI systems are transparent, explainable, accountable, and supported by risk mitigation guardrails. This could mean, for example, that businesses must provide disclosures of how consumer data is used and obtain explicit consent before using their data. Regulators can also require companies to meet risk assessment or audit requirements before and after deploying AI systems.
An example of this kind of regulation, the EU’s General Data Protection Regulation (GDPR) provides a legal framework for data protection and privacy. This legal framework is key to mitigating the privacy risks, malicious use, and security threats of generative AI.
The EU is going further and developing leading AI regulation, which includes audit requirements, copyright rules, and other requirements with generative AI in mind. The US has seen legislators introduce generative AI-specific regulations while China has passed a law specifically to regulate deepfakes, a kind of generative AI.
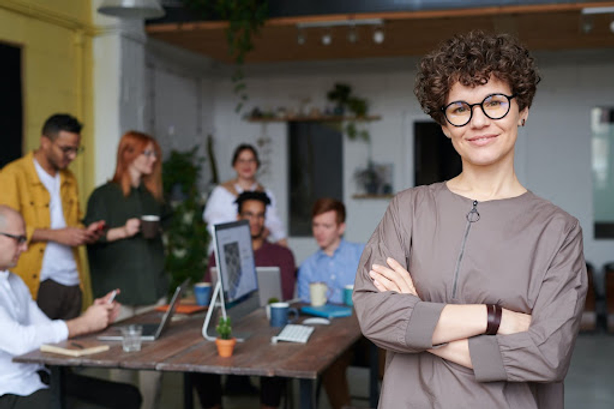
How the RAI Institute Helps
Generative AI is a rapidly advancing technology that provides both incredible benefits for businesses but also carries significant risks, like privacy risks and security threats.
To fully harness the benefits of this technology, it’s crucial to mitigate these risks and avoid the costs of lost revenue, lost customers, and legal fees. Research shows the benefits of operationalizing this risk management approach in an enterprise-wide framework. To set your business up for success with generative AI, you will need a strategy for internal generative AI use and generative AI sales to protect your business and build trust with your consumers.
But figuring out how to do this in practice is easier said than done. The Responsible AI Institute offers the support you need from AI experts – a Generative AI Policy customized for your business needs and objectives. Based on our industry-leading Responsible AI Implementation Framework, we offer our members smart Generative AI guidelines related to corporate best practices, principles, staff training, privacy, liability, all based on cutting-edge best practices, standards, and regulations. We inform our work through our industry-focused Generative AI consortiums.
We’ll take the guesswork out of what it means to be responsible when it comes to generative AI as well as other kinds of AI in this critical moment.
Interested in becoming a member and building out your business’s Generative AI policy? Learn more.
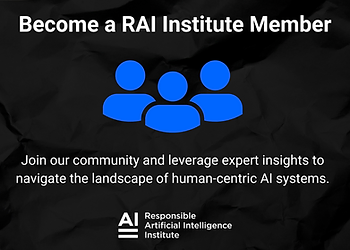
About RAI Institute
Founded in 2016, Responsible AI Institute (RAI Institute) is a global and member-driven non-profit dedicated to enabling successful responsible AI efforts in organizations. We accelerate and simplify responsible AI adoption by providing our members with AI conformity assessments, benchmarks and certifications that are closely aligned with global standards and emerging regulations.
Members include leading companies such as Amazon Web Services, Boston Consulting Group, KPMG, ATB Financial and many others dedicated to bringing responsible AI to all industry sectors.
Media Contact
For all media inquiries please refer to Head of Marketing & Engagement, Nicole McCaffrey, nicole@responsible.ai.
+1 440.785.3588.
Social Media