By Lucy He, RAI Institute Fellow
December 19, 2023
In 2023, we witnessed a rapid rise in Large Language Model (LLM) development and application. In this post, I will share some digestible knowledge on the state of current large language models, with a particular focus on the commercial landscape, safety research, and policy developments.
Mainstream LLMs include both proprietary closed source models (eg. OpenAI’s GPT, Google’s Gemini, Anthropic’s Claude) and open source models (eg. LLaMA, LLaMA-2, Vicuna, Pythia, Falcon). Some LLMs are easily accessible via chatbot such as Chat GPT, Llama Chat, and Claude. They have also been incorporated into search engines, such as Microsoft Bing and Google Bard, which allows for creative, balanced, and precise styles in its AI-powered search.
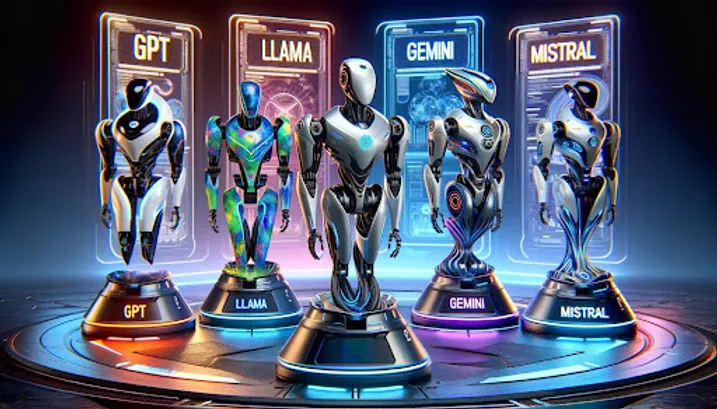
Contests among LLMs, image generated by DALL-E
The downstream application and specialization capabilities of these frontier models continue to expand at an accelerated pace. The developer API for GPT models, empowered by its expanded context window (128k for GPT-4 Turbo) and improved functional calling (which essentially allows GPT to manage API tools via APIs based on users’ natural language input), can significantly scale up and streamline its downstream use cases. In addition, the November updates from OpenAI also highlights multimodality usage, such as large scale experiments requiring the model to understand and digest visual output. It also incorporates the image-generation AI DALL- E into the system, further enhancing its capabilities.
The most recent entrant is Google’s Gemini, which was released 8.5 months after GPT-4. Gemini is built from the ground up for multimodality, meaning it is pre-trained from the start on different modalities (text, image, video, code, and others) instead of training separate components for different modalities. It is also optimized for different sizes. As of mid-December, the first version of Gemini Pro is now accessible via the Gemini API. While benchmark comparisons released by companies provide some hints on how these models compare, evaluation is still a quite ambiguous task in general.
On the open-source development side, Mistral AI and Meta are two leading players in providing open-source large language models. The Zephyr series models, derived from Mistral AI’s Mistral 7B and fine-tuned on conversation dataset, has performance that rivals that of Chat Llama 70B. Developers and researchers have also been fine-tuning models for specific down-stream usage, as well as testing its performance on various robustness and safety objectives. However, these studies are often inherently limited. Those outside of the companies who developed these models usually do not have the same level of compute resources or access to training data.
The multifaceted growth in LLMs is shaping a dynamic landscape in both proprietary and open-source domains.
Alignment research
The profound impact of LLMs on work and life necessitates rigorous research into their safety and alignment with human values, aiming to minimize unintended harm during their application. Researchers from both academia and the industry have been active in studying this topic. It is more feasible to conduct more thorough evaluation on open-source models like Llama, which researchers could easily obtain weights to, compared to closed-source proprietary models.
Since training is time and resource intensive and cannot easily be redone, researchers and practitioners have asked what the best way is to mitigate the potentially unsafe features already learned by the LLMs. Typically, a specific set of data (much smaller than the training dataset) is used for alignment.
One common approach is to fine-tune the model in order to instruct specific behaviors, which has been discussed at length elsewhere.
Another approach is reinforcement learning from human feedback (RLHF), which seeks to improve LLM alignment by incorporating a reward model that encodes human preferences when given pairs of output. For example, a human would prefer the model to refrain from answering rather than giving explicit instructions in response to a prompt asking how to make a bomb. These are mechanisms that help the model learn behaviors such as concealing private information and avoiding harmful comments.
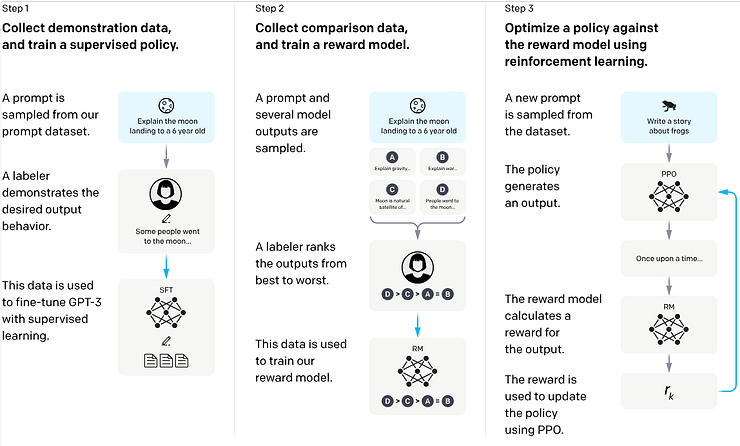
OpenAI illustration of RLHF
Redteaming and adversarial attacks (or ‘jailbreaking’) have also drawn a lot of focus in the research community. In a nutshell, these efforts seek to uncover failure cases in LLMs by either actively developing techniques that allow language models to break the safety guardrails, or by including red teamers who are ordinary users to bring valuable perspectives on harms that regular users might encounter (Microsoft Post, Hugging Face Post). For example, does appending certain prefixes or suffixes trick the model into bypassing the safety guardrails and telling the user whatever the prompt asks for? The value of these adversarial attacks is to guide iterations and improvements of LLMs to make sure that they are not susceptible to these attempts that elicit unsafe behaviors.
Policy
This month, the EU has finally reached agreement on its long-awaited AI Act, though the details of the text are still being worked on. The compromise text will subject foundational models with systemic risks to red teaming, risk management, and security reporting requirements. The progress on the AI Act comes on the heels of the Biden Administration’s October 2023 executive order emphasizing Safe, Secure, and Trustworthy Artificial Intelligence, which required – rather than encouraged – large AI players to share their safety tests results. It seeks to cover a comprehensive set of goals ranging from AI privacy to procurement, yet the problem remains that the order is quite broad and it is unclear how the review process will be calibrated across different AI use cases during implementation. There is also criticism from the tech community that such orders would stifle new competitors while significantly expanding the power of the federal government in the technical innovation field.
Parallel to these developments, industry leaders are proactively shaping the discourse, bringing more first-hand technical expertise to the policy discussions. In July, Anthropic, Google, Microsoft and OpenAI launched the Frontier Model Forum, an industry community focused on ensuring safe and responsible development of frontier AI models, aiming to develop safety standards ahead of policymakers.
At a high level, it appears that the alignment problem is not only a technical one, as summarized in earlier parts of the post, but also a key issue in the regulatory arena. A November policy brief from Stanford University Human-Centered Artificial Intelligence shed light on this “regulatory misalignment” problem by considering the technical and institutional feasibility of four commonly proposed AI regulatory regimes, namely mandatory disclosure, registration, licensing, and auditing. It argues against the formation of a super-regulatory body for AI, given the technology’s vast scope. It also cautions that policymakers should not expect uniform implementation of policies given the intrinsic ambiguity or even feasibility in operationalizing some high-level definitions like “dangerous capabilities” and “fairness”.
These are some of the commercial, alignment research, and policy themes that will shape the AI ecosystem in 2024.
Stanford Safe, Secure, and Trustworthy AI EO 14110 Tracker
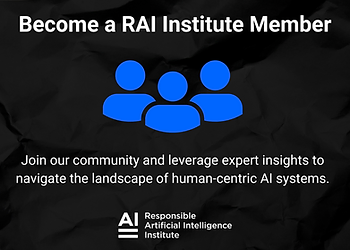
About Responsible AI Institute (RAI Institute)
Founded in 2016, Responsible AI Institute (RAI Institute) is a global and member-driven non-profit dedicated to enabling successful responsible AI efforts in organizations. RAI Institute’s conformity assessments and certifications for AI systems support practitioners as they navigate the complex landscape of AI products. Members include leading companies such as Amazon Web Services, Boston Consulting Group, ATB Financial and many others dedicated to bringing responsible AI to all industry sectors.
Media Contacts
Audrey Briers
Bhava Communications for RAI Institute
+1 (858) 314-9208
Nicole McCaffrey
Head of Marketing, RAI Institute
+1 (440) 785-3588
Follow RAI Institute on Social Media
X (formerly Twitter)