Artificial Intelligence (AI) is one of the most promising yet challenging issues of the modern era. As its adoption expands, so does the responsibility to ensure that the impacts of this exciting technology on people and the planet remain equitable, diverse, and inclusive.
To truly harness AI’s potential, we must intertwine its use with a robust commitment to DEI and Responsible AI (RAI), ensuring we don’t simply create ‘intelligent’ machines, but also foster a just and inclusive digital future.
The Current Landscape
AI’s growth is meteoric given the global demand for Generative AI. While it has significant potential, there’s a lingering shadow — a mirroring of societal inequalities that, if left unchecked, may amplify them.
So, what are the sources of these biases? Bias in AI, in particular, is critical to mitigate for the exact same reason it’s so powerful: AI’s power to vastly replicate human decisioning on a wide scale. With AI, suddenly, a small source of bias in a labeled dataset can be magnified across products and countries, affecting a person’s ability to access equitable healthcare treatment simply due to the color of their skin, for example. These impacts are disproportionately borne by people of minority or marginalized backgrounds, such as Black women, people of color, LGBTQ+ individuals, immigrants, etc.
It’s no surprise then that the severity of the problem of bias in AI and data related to AI is substantial.
The tech industry suffers an imbalance in representation with fairly homogenous groups developing AI systems. Over-representation of certain groups causes a consequent ripple effect that can deepen challenges for the underrepresented. Furthermore, broader societal factors create an imbalance in access to AI and their benefits, meaning that user feedback can be subject to the same biases. All of these factors can lead to skewed product design, blind spots, false assumptions, and thus, bias introduced into or perpetuated by the AI system.
Systematic oppression can also be replicated in the training data of AI systems, such as facial recognition systems that are trained on non-representative datasets that are over 85 percent white and 75 percent male. Due to this kind of unchecked bias, researchers often find bias in the dataset of “facts” used by AI.
In addition to the costs to humans and the planet, AI bias is costly for businesses as well. Recent research from DataRobot’s State of AI Bias Report shows that over 1 in 3 businesses have suffered an impact due to AI bias, such as lost revenue, lost customers, legal fees, and regulatory fines.
At the same time, to address AI’s negative impacts, there has been an increase in demand for regulation to curb the harmful or biased effects of AI, which presents significant reporting, auditing, and accountability responsibilities for businesses.
For example, in the U.S., New York City’s Local Law 144 requires that organizations using an automated employment decision tool perform a “bias audit” within one year of the system’s launch.
The draft EU AI Act, the most significant horizontal AI regulation to date, requires that businesses that develop “high-risk” AI systems assess the risk of their systems, which includes the risk of bias, mitigate that risk, and comply with a conformity assessment or set of controls related to the system’s logging capabilities, bias, fairness, human oversight, data governance, cybersecurity, and robustness of the model.
But, it’s not too late to explore deepening an organization’s RAI practice. Recent research from BCG and the Sloan Management Review reports that companies still have time to make responsible AI a powerful and integral capability beyond simple risk management. Leaders in RAI have the opportunity to make use of AI’s functionality with the assurance that their organizational practices related to AI match their organizational values and industry standards.
Conversely, without embedding Diversity, Equity, and Inclusion (DEI) and Responsible AI (RAI) principles in AI governance, organizations risk exacerbating global disparities. Simply put, Responsible AI governance, which includes DEI frameworks, is no longer optional for organizations seeking to harness the potential of AI today. Our aim? Building a digital future that isn’t just smart but is also just, equitable, and inclusive.
Understanding DEI in AI
Diversity, Equity, and Inclusion (DEI) refers to the policies and programs that ensure representation and participation from varied groups, covering age, race, gender, religion, culture, and more. Research and standards from notable institutions, such as OECD, UNESCO, IEEE, ISO, NIST, and more, reinforce the importance of these principles, especially in the realm of AI.
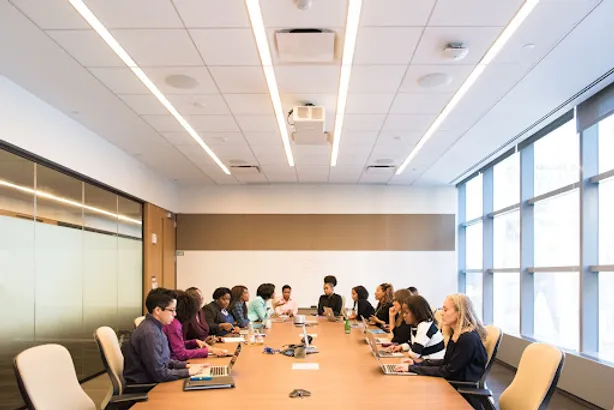
Implementing DEI and RAI: Key Measures
Ensuring AI operates within a responsible, equitable, and inclusive framework isn’t a passive process. It requires buy-in and consistent efforts but is well worth the investment.
Here are some of the ways organizations can do this well:
- Establish a Responsible AI Review Board: As part of an organization’s RAI implementation strategy, this Board should coordinate with executive and team levels and include external experts to ensure unbiased oversight.
- Diversify AI Teams: Representation and inclusion matters. Assembling diverse RAI boards, RAI officers, and system teams across the AI lifecycle is imperative.
- Integrate RAI and DEI Strategies: Define and link AI principles with DEI/ESG governance and training through enterprise-wide bias definitions, guidelines, and knowledge-sharing. Governance should include gates through the AI system life cycle, impact assessments, harm identification processes, and independent reviews.
- Prioritize Data Integrity: Data management best practices should be enforced throughout the AI system life cycle with measurement and evaluation related to data quality, representativeness, completeness, bias, and risk. Failure to include do so can lead to biased or inaccurate results, that can have serious impacts on a person’s health, employment and more, depending on the use case.
- Prioritize AI System Assessments, Audits and Certification: Establish regular audits to oversee AI performance and identify biases as well as self-assessments, vendor assessments and independent certification of AI systems. Research underscores the importance of third-party expert reviews in aligning systems with best practices, standards, and regulations.
- Plan for Labor Displacement: AI can displace jobs. It’s essential to upskill employees and address labor displacement, which could disproportionately affect workers from marginalized backgrounds.
- Mandatory DEI Training: Experts recommend that this training should be regular, comprehensive, and tailored to the role and organizational context, accompanied by processes to track, improve, and evaluate DEI training. Third-party-facilitated training is researched, vetted, and aligned with the specific DEI context of the organization and its training needs.
- Mandatory RAI Training: Organizations should regularly update teams on debiasing methods, concepts, and best practices as well as ethical and sociopolitical implications of the AI system.
- Notify Users: Transparency about AI’s involvement enhances trust and is a core part of current AI regulatory approaches so proper user notification—communications that are clear, relevant, and concise regarding the system’s impacts, use, and decision-making—are a must. These efforts might include public notice of intent to deploy an AI system with a justification and performance reporting requirements such as model cards.
- Engage Stakeholders Meaningfully: An inclusive dialogue with AI experts specializing in DEI, social justice, and community impacts ensures transparency and accountability. Engaging directly is more impactful than generic surveys.
- Offer System Recourse: Users should have multiple channels to report AI system errors or design flaws and seek solutions, similar to vehicle recalls. Recourse options include automated incident response protocols, taking the system offline to correct issues, providing user and data subject notifications, and allowing users to report adverse effects, which are all mentioned in AI risk management literature as effective recourse mechanisms. Furthermore, transparency in sharing changes made in response to errors, biases, or flaws builds trust in the AI system.
- Prioritize Continuous Learning: Proactively track AI regulations and RAI best practices across all operational jurisdictions. Embed processes for consistent testing and evaluation for bias-mitigatory performance.
- Community Collaboration: Work on mitigation measures with communities impacted by AI systems and contribute to relevant standards and open-source technology research to increase accessibility and collaboration.
Final Thoughts
Embracing DEI and RAI is imperative. The above suggestions can help begin the journey, but are just a start. To delve deeper, we recommend exploring the work of RAI scholars, especially those from marginalized backgrounds with DEI expertise.
At the Responsible AI Institute, we remain committed to shaping AI in a manner that’s beneficial and accountable to society at large while informed by how AI affects the most marginalized members of society.
In each of our Responsible AI Assessments, the implementation of DEI is a key control for measuring the responsibility of an organization’s approach to AI. We utilize leading research and standards to assess the depth of an organization’s efforts in operationalizing DEI principles, training, types of AI bias, equitable stakeholder engagement, and more.
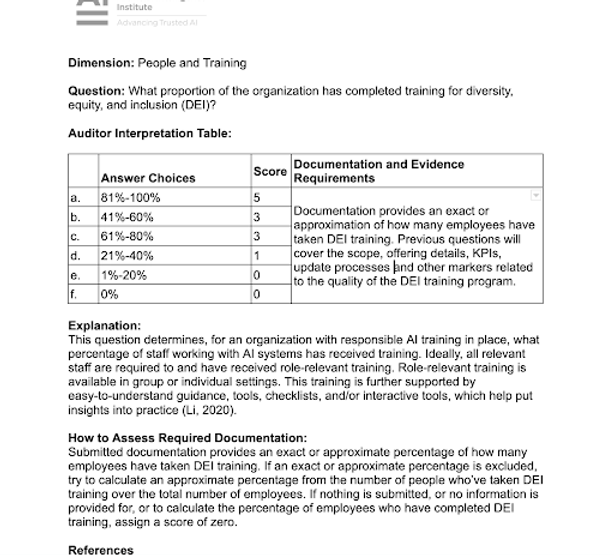
Sample question related to DEI in the Responsible AI Institute System-Level Assessment.
You can read more about the background and requirements for the RAI Institute’s Responsible AI Assessment Program in our Guidebook here. It contains an overview of the processes for our Assessment development, amendment, governance, and organization- and auditor-specific information related to certification and recertification.
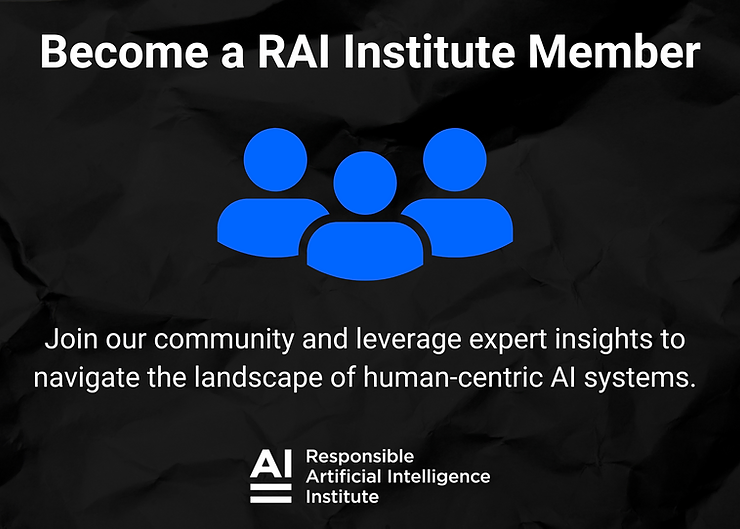
About The RAI Institute
The Responsible AI Institute (RAI Institute) is a global and member-driven non-profit dedicated to enabling successful responsible AI efforts in organizations. The RAI Institute’s conformity assessments and certifications for AI systems support practitioners as they navigate the complex landscape of AI products.
Media Contact
For all media inquiries, please refer to Head of Marketing & Engagement, Nicole McCaffrey, nicole@responsible.ai.
+1 440.785.3588.